The PDF version of a recent commentary in Nature (with a list of more than 800 signatories) has the provocative title “Retire statistical significance” and has been widely interpreted as a call for scientists to “rise up” against the concept of statistical significance. Closer reading of the commentary suggests that the main message of the paper is a call to stop the use of P values or confidence intervals in a categorical or binary sense, in order to be absolute as to whether a result supports or refutes a scientific hypothesis. This remains a radical proposal but perhaps does not signal the end for statistical tests or P values in biomedical research just yet.
The P value particularly, is a subject of much criticism and new approaches have been suggested by a number of commentators to replace it. However, until any of these alternatives gain widespread adoption, researchers are left having to make individual decisions about how to react to this criticism. For pharmacologists, particularly those who wish to publish in the British Journal of Pharmacology (BJP), the proposals in Amrhein et al are a problem. They appear to directly contradict advice given in the guidelines for publication in the BJP, introduced by Curtis et al, namely: “when comparing groups, a level of probability (P) deemed to constitute the threshold for statistical significance should be defined in Methods, and not varied later in Results (by presentation of multiple levels of significance).” In other words, statistical tests must produce a categorical outcome based on a P value of a defined threshold (normally as P = 0.05, or a 95% confidence interval) for all data sets in the paper. Frustratingly, every journal seems to have a different approach (see Figure 1). For example, Molecular Pharmacology (Mol Pharm) and also the Journal of Pharmacology and Experimental Therapeutics (JPET) supports varying degrees of statistical significance by recommending a different number of asterisks depending on the P value. The Journal of Physiology (J Physiol), on the other hand, recommends stating the exact P value in each case.
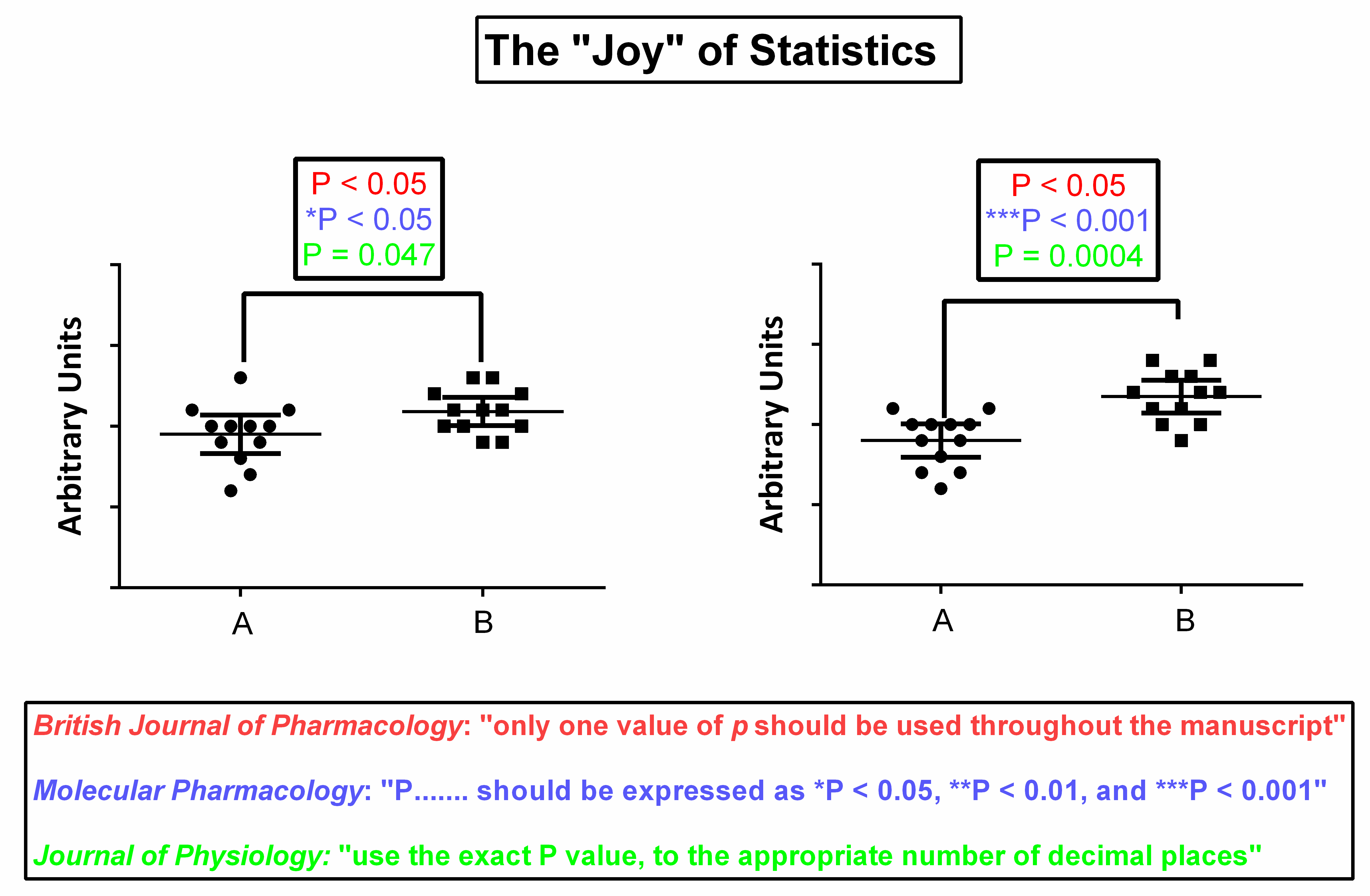
Figure 1. Different recommendations for the expression of statistical analyses can give different perceptions of the results obtained
So, what is correct? How should potential future authors in BJP and elsewhere approach this? In the spirit of the Amrhein et al article, I do not propose to make a binary choice here. After all, in the wider sense, all approaches seek to address the same issues of reliability and reproducibility in scientific research; issues which are particularly problematic in the area of biomedical science and thus pharmacology. The BJP approach is based around objectivity and removal of bias (whether unconscious or not). Here, decisions are largely taken away from the experimenter with a predefined statistical threshold coupled to a number of guidance statements around experimental design. There is much merit in this approach, and the journal does encourage authors to make appropriate caveats.
In truth, this is the approach that I have usually adopted throughout my career both in research and teaching. In research, I have usually tried to design experiments which give “either/or” answers and tried not to care too much, in advance, which answer materialises. In teaching basic statistics to second year BSc students, my approach to t tests, for example, has been to use a table to read off a critical “t” value for a known number of degrees of freedom and a chosen P value (almost always 0.05), then to calculate a t value from the data sets and compare the two to determine whether or not there is a significant difference between the data sets. This approach has always seemed both objective and satisfying. Often, however, this can be objectively, satisfyingly wrong. Inevitably, when such absolute, categorical decisions are made, P = 0.04 will take science in a different direction to P = 0.06. As Colquhoun and others have shown, much too often this will be the wrong direction.
The issue is illustrated by Figure 1, where the two graphs each display two simulated data sets plotted as both the individual data points and mean ± 95% confidence intervals. By eye, the data sets on the right-hand graph are more convincingly different from each other than those on the left. However the binary approach recommended by BJP (and the approach I would have used until now) shows that both differences are significant at the P < 0.05 level using an unpaired two-tailed t test (GraphPad Prism 6), and so, for interpretation and discussion, both differences are “real”. The more nuanced statistical approaches suggested by Mol Pharm and J Physiol give different degrees of significance (different degrees of certainty in the decision reached, perhaps) and naturally allow for a more nuanced interpretation and discussion. In this regard, it is of interest that a non-parametric Mann-Whitney test applied to the data on the left-hand side gives a P value of 0.062, above the 0.05 level of significance.
For this reason, I am persuaded by the Amrhein et al proposals, but I am not yet ready to abandon P values completely and, to my mind, the proposals come with at least two requirements. One of these requirements is data transparency and availability. If authors do not provide a statement about statistical significance, it is incumbent on them to make their data freely available so that others, particularly those researchers working closely in the field, can study the data in detail in order to support or refute the messages of the paper, ideally, perhaps, in the form of post-publication peer review. A second requirement is trust. In the absence of a statistical significance rule book or convention (however flawed), authors must provide a subjective narrative around the results and readers must expect that they can trust this narrative to be both informed and unbiased. However transparent and available the underlying data are, most readers will rely on the authors to guide their understanding and interpretation of the research. In an environment where “researchers’ careers depend more on publishing results with ‘impact’, than on publishing results that are correct”, this is surely the big challenge.
Going forward, I recommend a move away from binary or categorical decision-making to a more nuanced approach. I will take this opportunity to apologise to the many thesis-writers who I have criticised for doing the latter over the years: “What do you mean by “highly” significant? It either is or it isn’t significant”. I take comfort that, apparently, “only the strongest people have the pluck to change their minds, and say so, if they see they have been wrong in their ideas.” (Enid Blyton: The Naughtiest Girl in School).
This article is an extension of an earlier “Hot Topics” blog on the Guide to Pharmacology website.
Comments
If you are a British Pharmacological Society member, please
sign in to post comments.